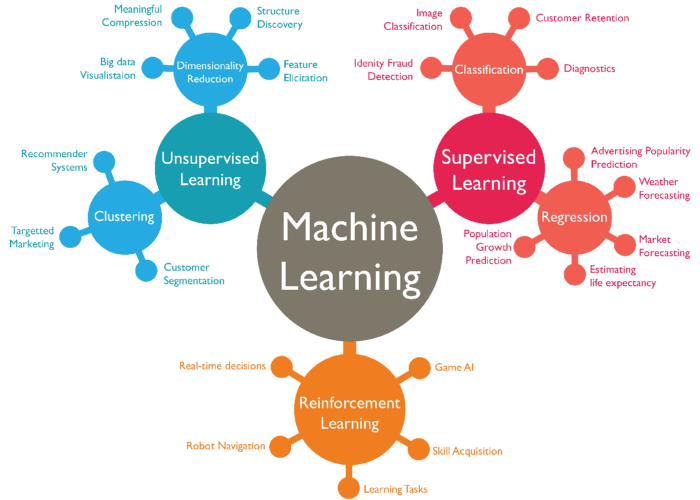
Originally posted on the KantanMT blog on August 31, 2021
KantanAI, alongside our NTEU Consortium partners Pangeanic and Tilde, has completed a substantial data upload to ELRC, with neural MT engines available for European Public Administrations via the European Language Grid.
The NTEU project goals were the gathering and re-use of the language resources of several European CEF projects to create near-human quality machine translation engines for use by Public Administrations by EU Member States. This massive engine-building endeavor encompassed every possible combination among EU official languages, ranging from high resource languages, such as English into Spanish, to low-resource languages, like Latvian into Maltese.
Each engine has been tested using the project’s specific evaluation tool MTET (Machine Translation Evaluation Tool). This tool was specifically developed for the project to rank the performance of direct combination engines (i.e., not “pivoting” through English) against a set of free online engines. Each engine was ranked by two evaluators in order to normalise human judgement and assess how close the engines’ output was to a reference human expression.

As we can see below, some language combinations (Irish Gaelic into Greek) were a challenge!

To avoid bias, human evaluators were unaware which input came from the NTEU engines and which input came from the generic, online MT provider that was used as benchmark. They ranked each input by moving a slider from right to left, ranging from 0 to 100. The aim of the evaluation was to assess whether the machine-generated sentence adequately expressed the meaning contained in the source, that is, how close it was to a human translation.
Evaluation Criteria
One of the challenged faced was standardising human criteria. Different people may have different linguistic preferences which can affect sentence evaluation. Thus, it was important from the beginning to follow the same scoring guidelines.
To standardise criteria, Pangeanic, alongside the Barcelona Supercomputing Center, put together a guide in order to guarantee all evaluators followed the same scoring methods, regardless of the language pair.
Unlike SMT methods (based on BLEU scores), NMT needed to be ranked on accuracy, fluency, and terminology. Those three key items were defined as followed:
Accuracy: defined as a sentence containing the meaning of the original, even though synonyms may have been used.
Fluency: the grammatical correctness of the sentence (gender agreements, plural / singular, case declension, etc.)
Adequacy [Terminology]: the proper use of in-domain terms agreed by the client and the developer, that are for use in production but may not be standard or general terms (i.e., the specific jargon).
When ranking a sentence, the following weights were typically applied:
- Accuracy: 33%
- Fluency: 33%
- Adequacy [Terminology]: 33%
In general, human evaluators deducted from 5 to 10 points for every serious error. The evaluation was the result of applying these deductions.
For example, one evaluator might have found two accuracy errors in a sentence (some information was missing, and non-related additional information had been added). The evaluator then subtracted 5% for the small error and 20% for the serious error from the Accuracy total. If the evaluator additionally found a small fluency error, they could decide to additionally deduct another 5%, too.
“This milestone represents the culmination of two years’ hard work by each of the NTEU Consortium members; we are delighted with the results of this project, and excited to observe how it might benefit European Public Administrations in the future.” Sinéad O’Gorman, Client Solutions Engineer at KantanAI.